Was das FZI besonders macht
Das FZI als Arbeitgeber
Du bist motiviert, etwas zu bewegen, brennst für Dein Thema und möchtest einen sinnvollen Beitrag für die Gesellschaft leisten? Dann gestalte mit uns am FZI gemeinsam die Zukunft! Unsere wissenschaftlichen Mitarbeiter*innen erforschen innovative Anwendungen für Wirtschaft und Gesellschaft auf international höchstem Niveau. Als gemeinnützige Einrichtung forschen wir ausschließlich zum Wohle der Gesellschaft.
Außerdem bieten wir Dir aIs Arbeitgeber die idealen Voraussetzungen für eine wirtschaftliche und akademische Karriere. Wir fördern Dich gezielt und unterstützen Dich dabei, Dein volles Potenzial zu entfalten.
In unserem Karriereportal findest Du alle Angebote für Mitarbeiterstellen, Jobs in Vollzeit oder Teilzeit, Abschlussarbeiten wie Masterarbeit oder Bachelorarbeit sowie unsere Ausschreibungen für ein Praktikum oder einen Hiwi-Job. Denn egal, ob Du als wissenschaftliche*r Mitarbeiter*in in den Forschungsbereichen in Karlsruhe und Berlin , als Mitarbeiter*in in den Zentralbereichen oder während Deines Studiums als Hilfskraft bei uns einsteigen willst: Du kannst Dich online auf alle Tätigkeiten bei uns direkt bewerben.
Du liebst Dein Thema?
– Sammle praktische Berufserfahrung
– Knüpfe wertvolle Industriekontakte
– Gründe ein Start-up
– Entwickle Dich persönlich weiter
Du möchtest etwas verändern und studierst:
Elektrotechnik – Mechatronik
Mathematik – Informationstechnik
Wirtschaftsingenieurwesen – Jura
...
Bewirb Dich jetzt bei uns
und werde Teil des FZI-Teams!
Arbeiten am FZI
Echte Zahlen, echte Mitarbeiter*innen, echte Begeisterung – lerne Deine zukünftigen Kolleg*innen kennen und wirf einen Blick in unseren Arbeitsalltag am FZI.
Echte Mitarbeiter*innen, echte Begeisterung – lerne Deine zukünftigen Kolleg*innen kennen und wirf einen Blick in unseren Arbeitsalltag am FZI.
*laut einer internen Umfrage
Interessante Projekte
Wir beschäftigen uns mit spannenden Themen aus der Informatik-Anwendungsforschung. Dabei arbeiten wir mit attraktiven Auftraggebern und Projektpartnern zusammen an topaktuellen Forschungsprojekten.
Im Video: Mitarbeitende des FZI
Motiviertes Team
Forschung ist Teamarbeit! Mit den motivierten Kolleg*innen am FZI macht die Zusammenarbeit gleich viel mehr Spaß. Wir tauschen uns bereichsübergreifend aus und stärken so unser fachliches Know-how.
Im Video: Wasilij Beskorovajnov, Abteilungsleiter am FZI
Angenehmes Arbeitsklima
Gutes Arbeitsklima
Am FZI herrscht eine lockere und angenehme Arbeitsatmosphäre. Wir gehen respektvoll und freundlich miteinander um und zwischendurch gibt es Raum für Vergnügen sowie das ein oder andere Späßchen im Team.
Im Video: Maria Baumann, Mitarbeiterin Communications am FZI
Kreatives Arbeiten
Den Satz „Das machen wir schon immer so“ wirst Du bei uns nicht hören. Am FZI sind wir offen für Neues und fördern nachhaltig eine Teamkultur, die Kreativität und Innovationskraft ermöglicht.
Im Video: J. Camilo Vasquez Tieck, wissenschaftlicher Mitarbeiter am FZI
Karriere mit Kind
Familienfreundlichkeit
Wir unterstützen unsere Mitarbeitenden in ihrer jeweiligen Arbeitsgestaltung. Eltern erhalten am FZI die Flexibilität, die sie benötigen, um Familie und Beruf gut miteinander in Einklang zu bringen.
Im Video: Dr.-Ing. Nicole Groß, Verwaltungsleiterin des FZI
Die Nähe zum KIT
Wir sind Innovationspartner des KIT. Unser Direktorium, bestehend aus 20 Professor*innen, begleitet unsere Forschung mit fachlich-wissenschaftlicher Exzellenz und unterstützt auch Dich bei Deiner Promotion.
Im Video: Prof. Dr. Jörn Müller-Quade, Direktor am FZI
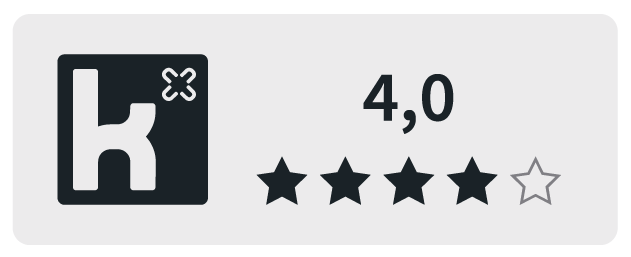
Feedback willkommen!
Auf der Arbeitgeberbewertungsplattform kununu haben uns Mitarbeitende, studentische Hilfskräfte, Aushilfen und externe Bewerbende bewertet.
Übrigens: Ein faires und wertschätzendes Miteinander ist uns am FZI sehr wichtig. In unseren Leitprinzipien erfährst Du mehr über unsere Werte, Verhaltensrichtlinien und Führungsleitlinien. Teil unseres offenen, respektvollen Umgangs miteinander ist außerdem die Duz-Kultur am FZI. Du kannst uns also gerne duzen!
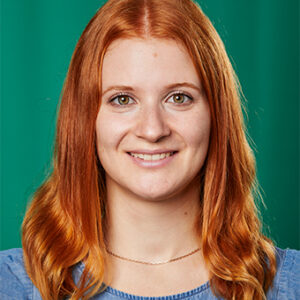

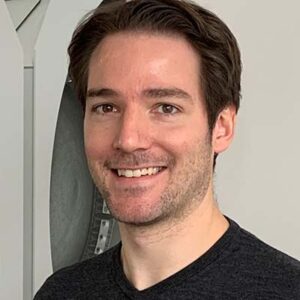
